
Weights & Biases (W&B)
The Weights & Biases platform helps streamline ML workflows.
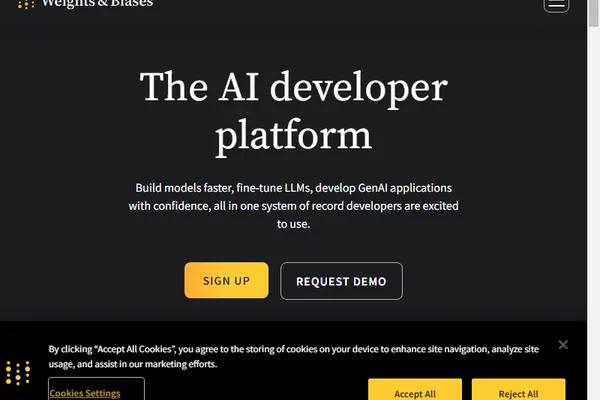
Pricing
Freemiumfrom $50/mo
Tool Info
Rating: N/A (0 reviews)
Date Added: May 9, 2024
Categories
Developer ToolsData Analytics
What is Weights & Biases (W&B)?
Weights & Biases (W&B) is a platform designed to streamline the machine learning (ML) workflow from end to end. It offers features such as experiment tracking, collaborative dashboards, artifact management, dataset and model versioning, interactive data visualization, hyperparameter optimization, and more. W&B aims to improve the efficiency and reproducibility of ML projects, making it easier to track and manage the entire ML lifecycle. It is trusted and used by leading ML teams worldwide.
Key Features and Benefits
- Experiment tracking that saves all relevant information for reproducing models later
- including the latest git commit
- hyperparameters
- model weights
- and test predictions.
- Collaborative dashboards for sharing and visualizing ML experiments and results.
- Artifact management for saving experiment files and datasets directly on W&B or storing pointers to external storage.
- Dataset and model versioning for easy tracking and management of different iterations.
- Interactive data visualization for exploring and analyzing ML data.
- Hyperparameter optimization to find the best set of hyperparameters for ML models.
- Automated ML workflows to streamline and automate repetitive tasks and processes.
- Model lifecycle management for tracking and managing ML models from development to deployment.
- Operational observability for monitoring and managing ML models in production.
- LLMOps and prompt engineering for facilitating the deployment and operationalization of ML models.
- Weave
- a suite of tools for developing and productionizing AI applications.
Use Cases
- Streamlining ML workflows and improving efficiency in ML projects.
- Reproducibility of ML experiments and models.
- Collaboration and knowledge sharing among ML teams.
- Managing and tracking datasets
- models
- and experiments.
- Visualizing and analyzing ML data.
- Finding optimal hyperparameters for ML models.
- Automating repetitive tasks in the ML workflow.
- Managing and deploying ML models in production.
- Monitoring and observability of ML models in production.
- Facilitating the deployment and operationalization of ML models.
- Developing and productionizing AI applications.
Loading reviews...